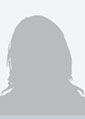
Seema Dhail
Bar-Ilan University
Israel
Title: QSAR Models for Cdk/Cyclin Inhibitors: A Comparison Between QM And Classical Descriptors
Biography
Biography: Seema Dhail
Abstract
Quantitative Structure Activity Relationship (QSAR) models correlate a specific activity for a set of compounds with their structure-derived descriptors by means of a mathematical model. Such models have been widely applied in many fields including chemistry, biology, and environmental sciences. The role of QSAR models in the identification of new compounds and in their subsequent optimization has been constantly growing and is now recognized by many practitioners of computer aided drug design methodologies. QSAR models highly depend on the molecular descriptors. Many types of descriptors with varying degrees of complexity have been described in the literature. These could be classified into 1-dimentional (1D), 2D and 3D or into "classical" and quantum mechanics (QM)-based. A rigorous comparison between the performances of classical and QM-based descriptors is currently lacking. Here we take the first step towards performing such a comparison by deriving QSAR models for a series of CDK/Cyclin inhibitors, important as potential anti-cancer therapeutics. We focused on a set of 40 ligands with known crystal structures (available in the PDB) and IC50 values. Reliance on crystal structures which are assumed to represent the bioactive conformations of these ligands allows for the accurate calculation of conformation-dependent 3D descriptors. Seven sets of molecular descriptors were calculated including 1D, 2D, 3D and QM-based and used to derive QSAR models by means of a Genetic Programming (GP) approach. Overall models with good prediction statistics were obtained highlighting the importance of specific descriptors